Statistical Inference for Stochastic Processes University of Sao Paulo
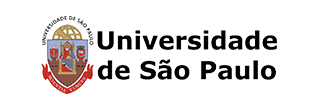
Course Overview
Stochastic processes are natural models for phenomena occurring in time and for spatial systems. Modeling natural phenomena using stochastic processes requires the knowledge of specific inferential and statistical model selection tools. Moreover, stochastic processes have also been used as computational tools in statistical inference, as exemplified by Monte-Carlo Markov chain algorithms for sampling probability distributions.
Learning Achievement
To present basic notions of statistical inference for some important classes of stochastic processes.
Competence
Course prerequisites
Grading Philosophy
Students will be evaluated through projects, seminars, exercise lists and write tests,
Course schedule
1) Statistical inference for Markov chains. Maximum likelihood estimation. Estimation of the order of the chain. 2) Statistical inference for stochastic chains with memory of variable length. The algorithm Context. 3) Context tree selection using the Bayesian Information Criterion (BIC). The algorithm_CTW. 4) Statistical inference for hidden Markov models. 5) Gibbs states. Interaction graph selection and maximum likelihood estimation for the_Ising_model. 6) Simulations using Monte-Carlo Markov chains (MCMC)._Glauber_dynamics, Gibbs sampler, Metropolis algorithm. 7) Perfect simulation algorithms.
Course type
Online Course Requirement
Instructor
Jefferson Antonio Galves, Florencia Graciela Leonardi
Other information
Site for Inquiry
Please inquire about the courses at the address below.
Email address: https://www.ime.usp.br/en